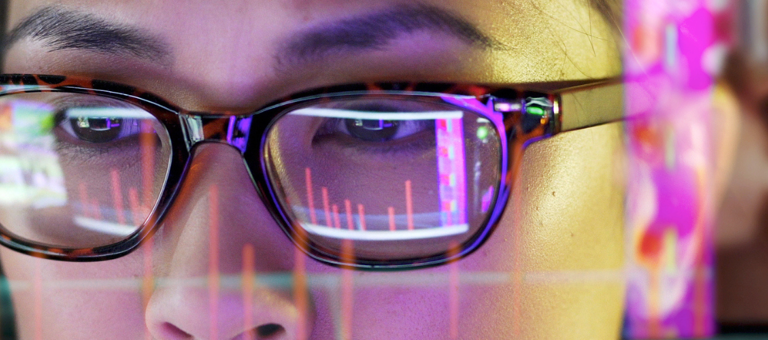
Worried about AI hallucinations? RAG system architecture is the secret to more value from AI investments
6 min read 3 October 2024
Gen-AI solutions are already supercharging productivity in many organisations, but as the technology develops, there’s more to come. Find out how new RAG system architecture can take productivity gains to the next level and discover key principles to consider as you plan your move.
Across sectors, Gen-AI solutions are helping employees work smarter and faster – retrieving relevant documents to answer complex queries at pace, and underpinning chatbots and conversational AI to automate responses to common customer queries. The productivity gains are impressive – take a look at the remarkable results AI is delivering for our client, Energy Networks Association, for example – but the answers generated by many current AI solutions are far from perfect. Sometimes the technology ‘hallucinates’ – doing its best to provide a useful response even if it doesn’t really have all the facts it needs. This may lead to bizarre-sounding answers that have no connection with reality. At other times, it can generate tone-deaf answers that fail to reflect the context in which a question is being asked. Hallucinations and discordance are holding back some organisations from pushing on with wider application of AI technology.
RAG system architecture: what is it and how does it work?
Retrieval Augmentation Generation (RAG) system architecture, with appropriate guardrails addresses the problem, giving organisations the confidence to expand the use of generative AI and maximise productivity gains. Solutions built around RAG deliver better answers than first-generation AI because they provide the generative model with additional, relevant information. At Baringa, we use RAG systems to ground our generative agents with additional contextual information, leveraging the efficiency and precision of retrieval systems with the creative and adaptive capabilities of generative models.
More reasons to choose RAG system architecture
RAG system architecture helps make the text generated by your AI solutions more accurate and relevant. There are plenty more reasons to use it, too:
- RAG systems are better at adapting to new data without extensive retraining because of their built-in access to an enhanced knowledge base
- They are easily scalable and leverage fast retrieval techniques, which makes them more efficient and robust when it comes to handling multiple queries
- RAG systems are customisable for specialised domains, such as HR and Procurement, through the curation of document sets
- They build trust by referencing specific documents used in the generation process, allowing users to trace the source of information
- RAG systems deliver an enhanced user experience by enabling more interactive and informative systems. This includes conversational agents that provide contextually appropriate responses by retrieving and synthesising information in real-time.
Key principles to maximise returns
Our teams are involved in architecting, building and deploying RAG systems for a range of clients, from large US telecommunications companies to a leading energy supplier. For example, for one company, we have developed a new HR Corporate Assistant that retrieves information from an enhanced knowledge base to generate better responses for employees using the tool, and we have built a central market intelligence platform that uses LLMs and agentic pathways to solve network questions.
For projects like these, we focus on several key principles to get the best from RAG system architecture:
- Design to realise modularity benefits: Our solutions give clients the opportunity to swap in the latest LLM releases and assess the viability of the model against their existing production baseline without disrupting overall architecture.
- Build for scalability: Making RAG systems a simple extension of existing cloud native maturity ensures their ability to handle growing data volumes, increased user demands and more complex processing without compromising performance or quality. Our teams have designed and built systems with all the major hyper-scalers (AWS, Azure, GCP).
- Target performance efficiency: Optimising capacity needs and managing token requirements effectively ensures the RAG systems we design handle large datasets and extensive token usage efficiently.
- Optimise security: Our solutions incorporate measures like rate limiting to prevent abuse and web application firewalls to block malicious traffic.
- Protect users: We deploy guardrails to set and implement boundaries on AI behaviours and ensure ethical and social expectations are met. We also use guardrails to prevent AI returning unexpected responses, thereby protecting users and ensuring data integrity.
Get in touch with Ed Sharkey or Kelly Hume to discover more about using RAG system architecture to get increased value from your AI investments.
Want to discover how Baringa can help you? Explore our Data and AI consulting services
Our Experts
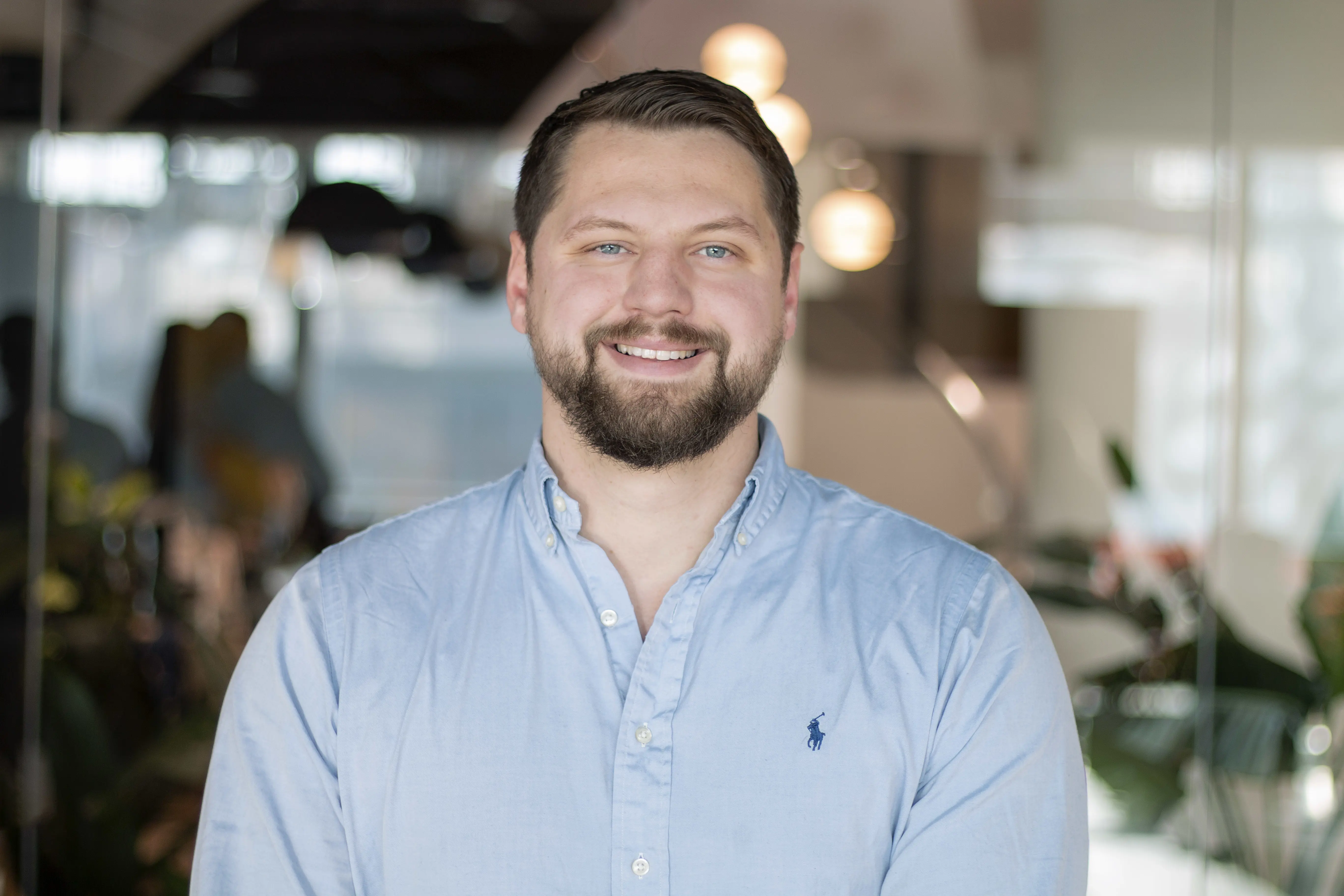
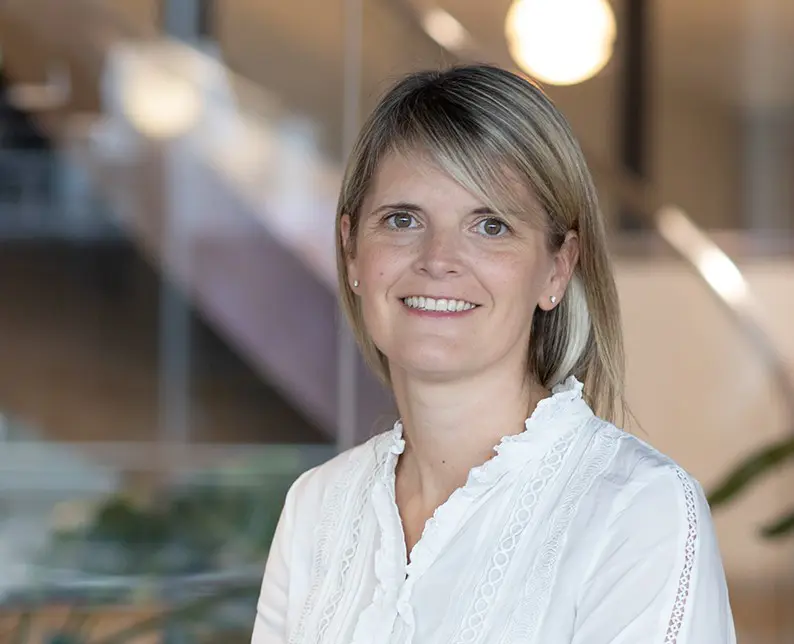
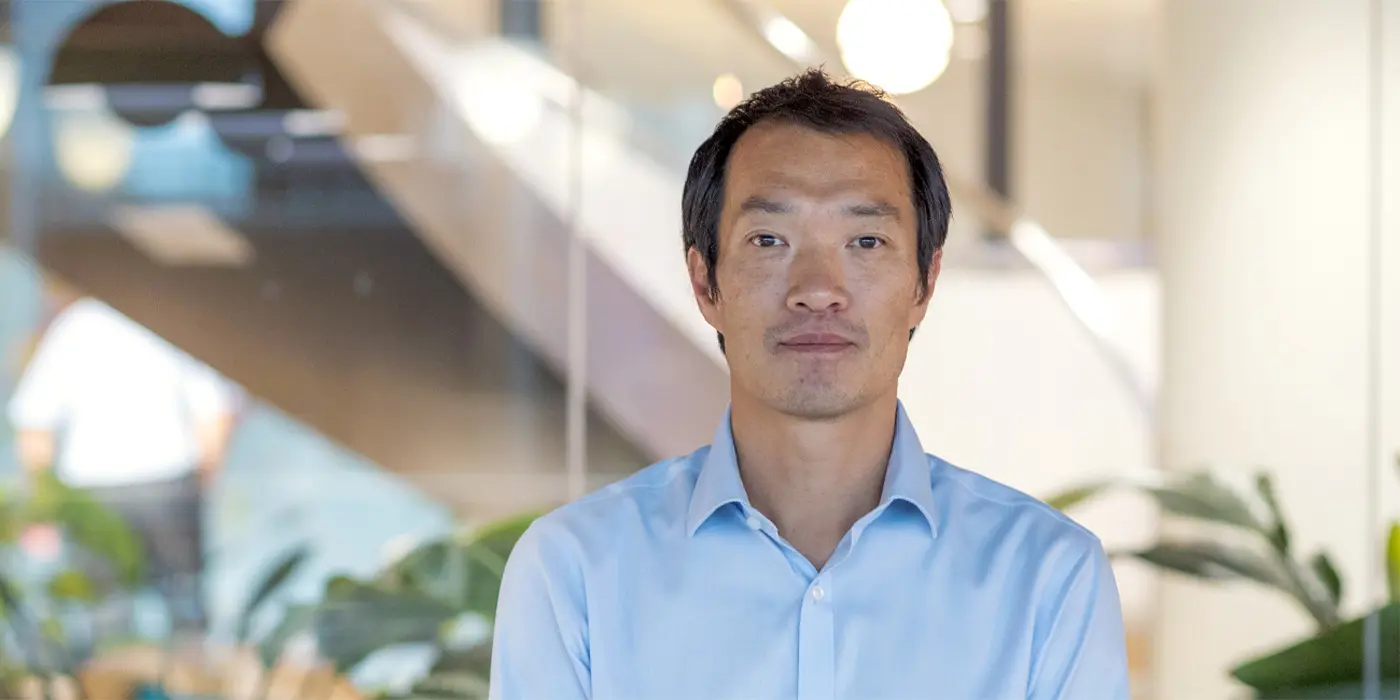
Related Insights
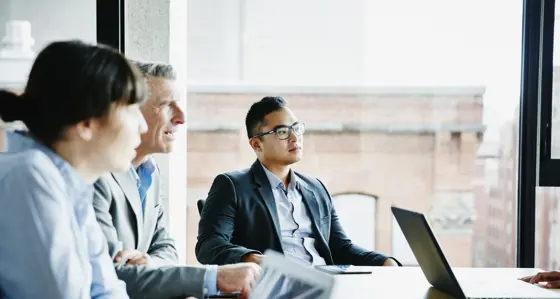
How do super CROs navigate the double-edged sword of AI?
Explore how AI is transforming risk management for superannuation funds and the strategies Chief Risk Officers are using to navigate the complexities of the modern financial landscape.
Read more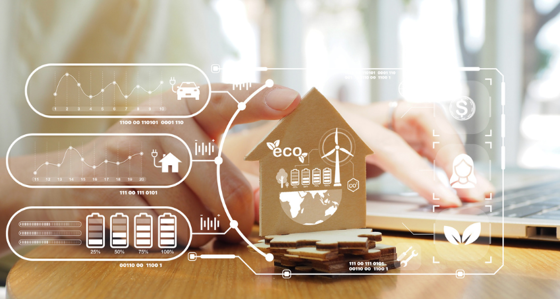
Data-driven decarbonisation: how analytics and AI are supporting the energy transition
Data and AI are key to enabling mass-market decarbonisation. From standardisation to sharing, everyone has a role in ensuring their approach delivers industry-wide benefits alongside individual competitive advantages.
Read more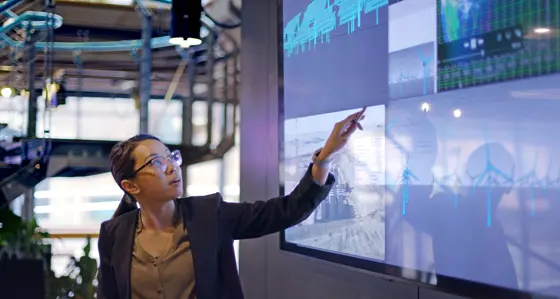
The role of digital technology in the energy transition
With so much at stake, we see four digital technologies standing out as key.
Read more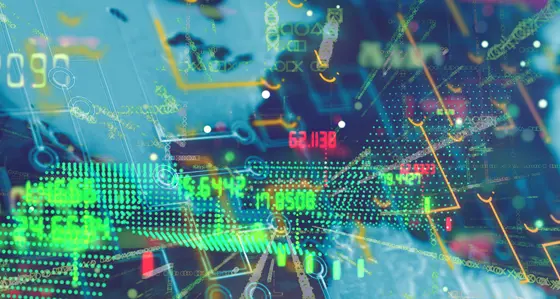
Leadership Dialogues: Data management and workflow solutions
In this video, Baringa's Lucine Tatulian speaks to Roy Saadon, CEO and Co-Founder of Access Fintech about data management and workflow solutions.
Read moreAre digital and AI delivering what your business needs?
Digital and AI can solve your toughest challenges and elevate your business performance. But success isn’t always straightforward. Where can you unlock opportunity? And what does it take to set the foundation for lasting success?