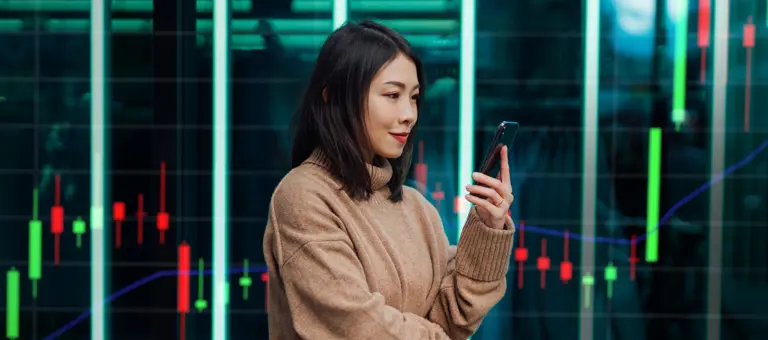
How do Asia’s commodity trading organisations become AI future-ready?
6 min read 1 December 2024
When I agreed to join a panel discussion at the FTLive Commodities Asia Summit on Integrating IoT, Big Data and AI to transform trading operations, I didn’t realise Singapore’s Minister for Trade and Industry would use his keynote at the event to announce the launch of two new AI Centres of Excellence. It was yet another signal that local commodity trading organisations have plenty of support and incentives to adopt emerging technologies, including AI, machine learning (ML) and advanced data analytics, with the potential to revolutionise the sector.
We are just starting to grasp the potential for AI, ML and analytics to revolutionise business operations – far beyond immediate use cases like automating repetitive tasks or optimising processes. Already, use cases specific to commodity trading include:
- Enhanced market analysis – The adoption of AI-driven predictive models has enabled the use of vast amounts of historical data and real-time information – weather patterns, geopolitical events, and market trends – to forecast commodity supply, demand and price movements.
- Algorithmic trading – Insights gained and patterns learnt can enable AI-powered trading systems to enable trades at high speed and precision, identifying more market inefficiencies and trends, giving traders a personalised competitive edge.
- Risk management – While today’s risk management processes focus on a few core areas of risk, AI can broaden traders’ ability to assess and prioritise risks from across different risk classes. We can imagine a future where executives receive an automated daily report of the top 10 risks for a trading business across all risk classes – not just market or credit risk.
And this is just the tip of the iceberg. Soon, exponential improvements in the technology will change how we look at sizing and evaluating what can be done with AI and ML. Given what we know today, it will be a brave executive who chooses to bet against these technologies.
But integrating them into existing legacy infrastructure remains a challenge. Many organisations are also questioning how they will maintain an edge in an industry that thrives on specialised access to information and the trading styles of individual traders. Harnessing AI and analytics requires surfacing valuable data and leveraging it at scale. Will traders be prepared to share their ‘secret sauce’?
If your organisation is starting to think about these issues, here are some areas to consider:
Understand AI, its limitations and intended applications
Companies struggle to identify which AI use cases to develop in-house and which to source as off-the-shelf solutions. Before you decide to build, make sure you’re aware of what’s already available. We see lots of instances where companies invest to build something they could have bought at a fraction of the price with very little functional difference.
Only build if it supports your competitive advantage. The consideration here is balancing customisation with cost and speed. Faster, lower-cost off-the-shelf solutions lend themselves to non-differentiating tasks, like pre-built solutions for forecasting prices or demand-supply scenarios. In contrast, it could be worth developing ring-fenced in-house solutions for use cases where traders need greater control over data usage and intellectual property, or where models can augment areas of unique organisational value – and there are no existing off-the-shelf products.
AI needs a data strategy – set up your data for success
A data strategy is essential to ensure information is available, useful, reliable and trustworthy. In most trading organisations, data is currently in walled gardens, with fundamental data, market data and trade data sitting in different silos. Trading businesses also depend on a high proportion of external data that is naturally federated and of different qualities, tenors and granularities.
The first step towards getting ready to use AI tools is to develop the ability to pull valuable data from all these diverse sources into a place where it can be accessed. This is hard to master initially. It needs to be done pragmatically over time as analytics, ML and AI tools are proven.
To keep costs down, consider a dual-speed data infrastructure: separating real-time needs versus batch or less time sensitive data. Understanding user needs, data flows and the inter-relation between data across multiple systems reduces both inefficiencies and increases agility when applying AI and analytics tools in the trading landscape.
It’s worth shifting from a monolithic data structure to a modular approach that inherently supports scalability. This is much easier than it would have been a few years ago because vendors now build APIs into tools that allow read/write access, reducing the cost and effort of integration. In future, prioritise architectures that allow for easy module additions, replacements or upgrades as technology evolves and new data sources become available.
Explore multiple entry points for AI adoption
Think about the menu of potential entry points for your organisation. As each organisation and user adoption is unique, we often see entry points that focus on driving operational cost savings and efficiency. We also encourage our clients to ask themselves how gathering new sources of market information could be performed enhanced, at scale and with real-time sensitivity analysis.
Choose areas where a human, no matter how talented, can only do so much. What if you apply an AI agent that can do as much of that task as you want at scale? What will your talented human now do with the information the AI agent sends them?
Consider the idea of trading agents. It may be a tough sell internally given traders often don’t want to share their secrets. But we see the opportunity to have personalised AI agents that may enable traders to replicate strategies across time zones or, with added information, enhance the style of a particular trader. Or, more simply, it could be about having a trainable copilot operating within an individual trader’s own environment, taking over AI-appropriate micro-tasks. To this point, we can imagine a future where personal AI agents:
- Summarise a trade by voice and draft the recap or capture trades into a what-if calculation engine to support deal negotiations.
- Help traders to analyse historical market patterns, responding to natural language questions like: “What was average the impact on my LNG Atlantic forward curve the last 10 times there was a major hurricane in Gulf of Mexico?”
- Help traders to quickly compare and contrast strategies, responding to instructions like: “Compare and contrast the time and cost impact of Suez versus the Cape of Good Hope route.”
Assemble the right team
People are critical to securing value from technology. Introducing AI-powered tools needs to be led by the business. The key is to define, empower and enable a hybrid, cross-functional team that spans your business and IT. Traders need to be involved in any AI development and use case socialisation. AI agents are standard, but their use and output requirements will be different for each trader and organisation.
Appoint someone to be accountable for bringing AI to the table in your organisation – to create a culture where data is treated like an asset that can be leveraged to create value. You need a champion to prompt teams to keep asking: “How can we use AI to solve this challenge?” You can start small with incremental changes based on specific use cases to focus organisational thinking and effort. Having someone pull together high-value ideas to share with leadership is a huge step forward.
How can Baringa help you get ready to use AI in your organisation?
The key is to build the foundations for AI and data incrementally, assembling trusted data and growing AI capabilities over time. At Baringa, we help our commodity trading clients to understand what good data management looks like and how to apply the right analytical tools, including AI, to release value. We understand how commodity traders operate and are experts in the systems and data architectures on which you depend. We can help you identify opportunities, develop business cases and set up sound data strategies. As independent advisors working with a variety of technology providers, we collaborate with you to unlock the benefits and then define and drive the path to AI in production.
Our Experts
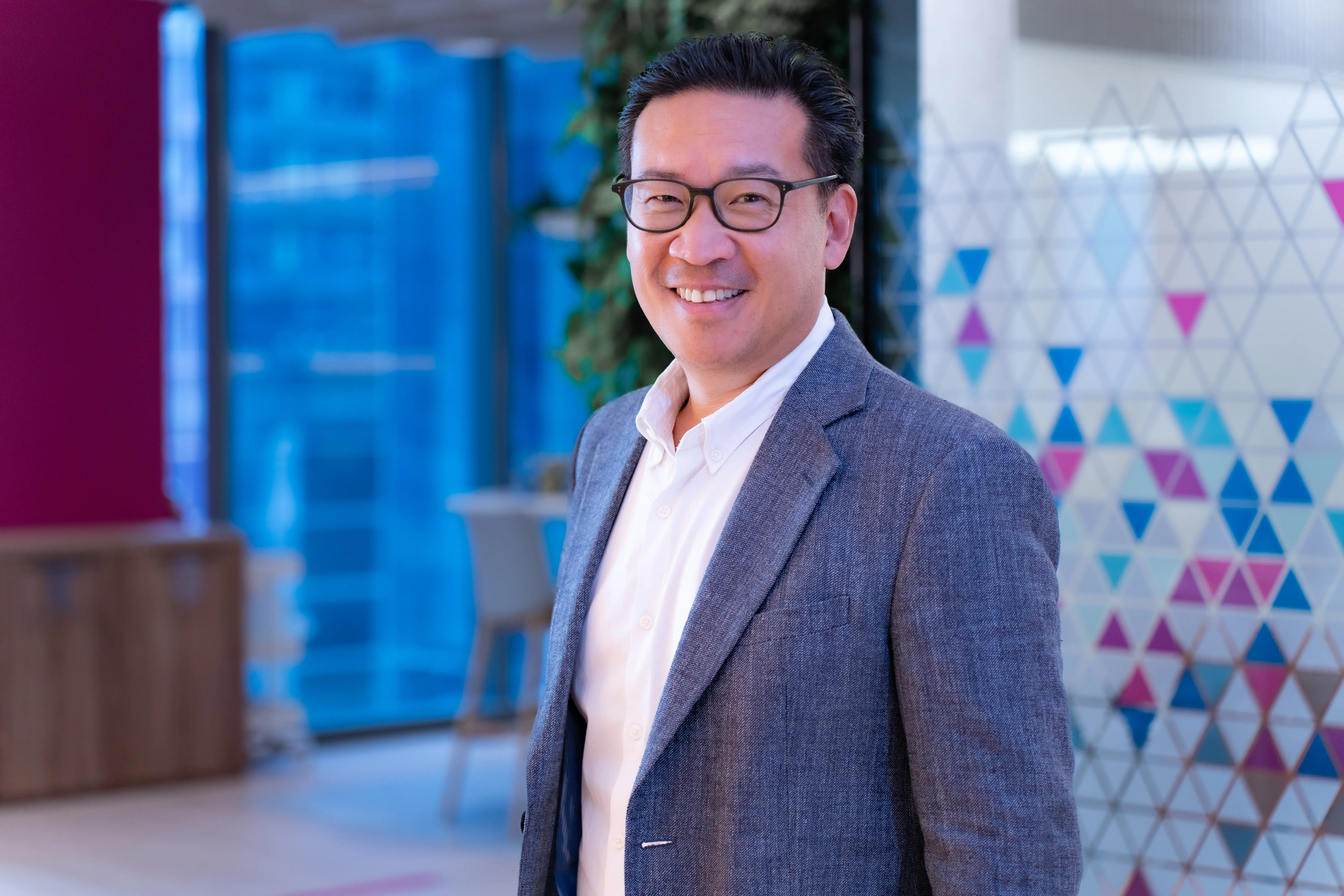
Related Insights
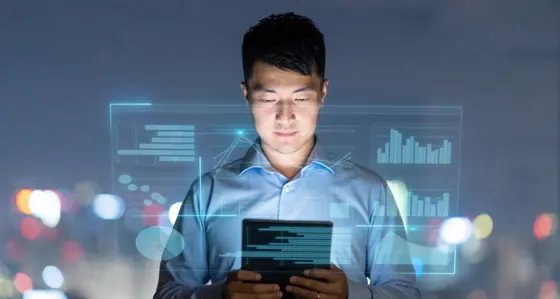
Organisational agility: a guide to taking the first steps
Our business agility and operational excellence expert Simon Tarbett offers some advice to clients when asked the question, Where To Start?
Read more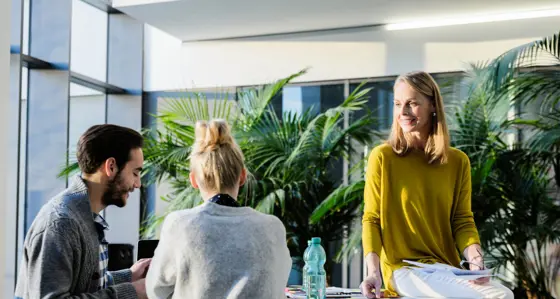
The need for agility to grow revenue and thrive in a turbulent market
We’re looking at how organisations need to be agile if they’re to grow revenue and thrive in a turbulent market.
Read more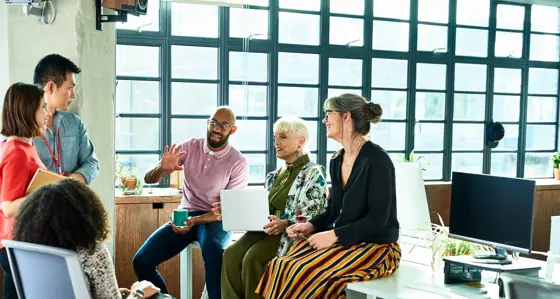
How to set targets to drive business performance
Targets are essential for driving better business results. Let’s discuss what companies should – and shouldn’t – be doing.
Read more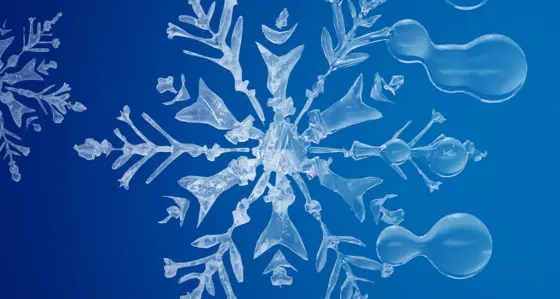
How frozen organisations can become fluid
To develop organisational agility, focus on being like water.
Read moreAre digital and AI delivering what your business needs?
Digital and AI can solve your toughest challenges and elevate your business performance. But success isn’t always straightforward. Where can you unlock opportunity? And what does it take to set the foundation for lasting success?