Artificial Intelligence (AI) is a transformative force in the financial services industry, revolutionizing operations, enhancing customer experiences, and driving innovation. However, as AI becomes increasingly integrated into financial institutions' processes, greater regulatory oversight has become inevitable.
With the rapid advancement of AI technology, concerns have been raised about potential risks and challenges associated with its deployment, prompting regulators to consider the need for AI-specific regulations.
As AI continues to reshape the financial services landscape, industry participants should proactively address the risks associated with its adoption. By doing so, financial institutions can build trust, ensure compliance, and harness the full potential of AI to drive sustainable growth and deliver value to their customers.
Increasing use of AI in financial services
With many financial institutions automating aspects of customer service through AI-powered tools, the resulting client segmentation must be fully considered and managed.
Highly personalized engagement can be delivered, while eliminating manual, low-value processes such as those related to Know Your Customer (KYC) procedures and credit scoring. These often use in-house analytics and AI-powered probabilistic tools to analyze a combination of factors such as existing relationships within a household, credit history, income, spending patterns, geolocation, and more.
While personalization offers tremendous benefits, client segmentation requires thorough risk management to avoid disparate treatment and unfair practices against a consumer, which can potentially violate Fair Lending laws and regulations along with privacy regulations including the Federal Trade Commission Act, state legislations such as the California Consumer Privacy Act, and the recently introduced American Privacy Rights Act (APRA).
Without adequate controls and human involvement pre- and post-output, undesirable behaviors and outcomes will be amplified since AI models can absorb biases from existing data. To mitigate and monitor this bias, financial institutions should take a closer look at how AI is developed, trained, deployed, and managed across the business throughout the entire AI development lifecycle while adopting a co-pilot operating model. Regularly conducting comprehensive audits and bias assessments is crucial in identifying and mitigating potential sources of bias in training data and algorithms, even if training data was collected with underrepresented populations to reduce bias. In parallel, data management including assurance, lineage, and provenance must be updated to ensure biases are understood and risks are managed.
Model outputs to regulatory bodies
The limited explainability of AI systems, known as the "black box effect", gives rise to a range of operational, compliance, and reputational risks. Regulatory bodies are placing increased emphasis on data governance policies within the financial sector to ensure the protection of data used by AI systems and to promote transparency in decision-making processes.
One of the primary concerns for financial institutions is the quality and privacy of training data, as the reliability and accuracy of AI models depend on various factors including input data. As regulatory expectations are still evolving, compliance remains a top priority for financial institutions. However, there are steps that firms can take to address the black box effect and move towards compliance.
To begin with, comprehensive documentation of the entire AI lifecycle should be transparently developed and maintained. This includes documenting the development processes, decision-making methodology, testing and validation procedures, and outcomes. Sensitivity and post-hoc analysis should be implemented to evaluate how AI models react to alterations within their output. Additionally, privacy and security must be ensured through robust data governance practices. Financial institutions need a thorough understanding of how enterprise data is acquired, used, and managed by AI systems.
Practitoners should be prepared to provide evidence and explanations to regulators so they can fully comprehend how AI systems are utilized internally. Defining and categorizing acceptable use cases and risks, as outlined in regulations such as the EU AI Act, can help in understanding the implications for privacy, controls, and the use of anonymized data before deploying AI models.
By implementing these methods, financial institutions can enhance their understanding of AI model decisions and make them more interpretable for both internal stakeholders and regulatory bodies. This approach fosters trust, accountability and explainability, paving the way for responsible and compliant use of AI in the financial industry.
As use cases rapidly expand, and organizations seek to incorporate the technology, they need to develop a holistic AI governance framework that considers strategic areas such as technology, data, and privacy.
Cyber implications of AI adoption
The financial sector is also hyper-sensitive to the cyber implications of AI developments such as costly cyber-enabled crimes. Traditional crimes faced by the sector such as phishing/spoofing, business email compromise, credit card fraud, identity theft and SIM swap are further exacerbated due to the adoption of AI by cybercriminals as they continue to adjust their tactics and techniques.
In the same manner, the financial market will experience similar risks when threats become empowered by AI. On May 6, 2010, the Flash Crash saw US stock indices, including S&P 500 and Dow Jones Industrial Average, tumble virtually 1,000 points in just minutes, erasing nearly $1 trillion in market value. While the crash was largely attributed to algorithmic trading by a single individual, vulnerabilities within AI trading algorithms still exist today and can be exploited by malicious actors to manipulate market conditions and undermine investor confidence at a larger scale. Similarly, software malfunctions and technical failures can erroneously execute trades that could trigger a chain reaction of financial losses and disruptions to market stability.
In 2023 alone, losses due to cyber-enabled crimes amounted to over $12.5 billion, representing a 21% increase from the year prior[1]. Note this amount only accounts for crimes reported by the victims, meaning the actual losses likely exceed the reported amount.
In the realm of cybersecurity, AI-powered tools have revolutionized the threat landscape, enabling cybercriminals to swiftly expand their operations and amplify the frequency of attacks. These advanced technologies not only facilitate scaling of malicious activities but also pose a challenge to conventional security incident detection systems – e.g., user behavior analytics, customer identity verification, and more – making it harder to identify and thwart cyber threats. As a result, the need for innovative security measures has become more pressing than ever before.
The emergence of advanced AI-powered tools and ease of access have given threat actors a new opportunity to quickly target organizations without proper AI governance, placing an increased priority on compliance in identifying, detecting, combating, and reporting crimes against existing regulatory obligations.
Regulatory landscape
The European Union (EU) continues to be the leader in the regulation of AI with the recent AI Act. This is a prescriptive set of AI regulations imposed across all stages of the development lifecycle.
The Act defines AI as: “machine-based systems designed to operate with varying levels of autonomy and that may exhibit adaptiveness after deployment and that, for explicit or implicit objectives, infers, from the input it receives, how to generate outputs such as predictions, content, recommendations, or decisions that can influence physical or virtual environments”.
The EU standardizes AI systems into various levels of risk that the EU has deemed to be permissible: minimal, limited, high, and unacceptable. This standardization fundamentally paved the way for organizations to begin thinking about and managing AI risk holistically.
In contrast, the UK, with its pro-innovation stance, took a different approach, evidenced by flexible regulations and governance aimed at addressing risks associated with AI while maintaining consumer confidence and trust.
On the US side, there is a significant level of activity regarding AI regulation, albeit still in its early phases, by regulatory authorities with several states forming AI-related task forces to consider implications around data and privacy. While states have expressed differing priorities, there are overlaps with existing guiding principles, such as the Department of Homeland Security’s Fair Information Practice Principles[2]. Guidance at the federal level comes via President Biden’s executive order on AI in 2023, with the White House’s announcement for all executive agencies to fulfill the 90-day actions assigned by the order.
On March 28, 2024, the Office of Management and Budget (OMB) at the White House issued its inaugural government-wide policy to address the potential risks associated with AI and leverage its advantages. Led by Vice President Kamala Harris, the new OMB policy directs several high-level actions that require completion by federal agencies, including addressing risks from the use of AI, expanding transparency of AI use, advancing responsible AI innovation, growing the AI workforce, and strengthening AI governance. This was a significant step, which in conjunction with President Biden's AI Executive Order, demonstrates a commitment to effectively manage AI technology and maximize its benefits[3].
Most recently on April 5, 2024, the US Congress released a draft of the American Privacy Rights Act (APRA), a federal privacy bill that includes provisions on data security, executive responsibility, minimization, and civil rights.
This came on the heels of an October 2023 subcommittee meeting on AI where Frank Pallone, Ranking Member of the House Energy and Commerce remarked: “I strongly believe that the bedrock of any AI regulation must be privacy legislation that includes data minimization and algorithmic accountability principles”. This highlighted the criticality of protecting consumer privacy posed by AI and minimizing existing data collection practices.
As an example, strict regulations would prohibit companies from utilizing individuals' personal information for discriminatory purposes[4]. This would grant individuals the right to opt out of a company's use of algorithms that make decisions pertaining to various aspects such as healthcare, housing, insurance, employment, credit, and more. Notably, this same bill would preempt state privacy laws.
It’s clear that as firms continue to widely embrace AI, federal guidance from the current administration is becoming more defined in parallel.
Baringa recommendations
Given the varying regulatory mandates spanning across many jurisdictions and the pace at which AI technology evolves, Baringa has developed a technology-agnostic, end-to-end approach to AI governance and control. This approach equips organizations with the tools to employ effective governance across all AI-related processes. It empowers them to leverage the full potential of this technology to further drive strategic imperatives. A sound AI risk management framework begins with the policies and procedures, definition of roles and responsibilities, which includes the board, a risk responsive culture, leveraging risk assessments and controls, and stakeholder engagement and training.
In our experience deploying AI at both public and private organizations, we have often encountered a nervousness about novel AI solutions. This is due to the fast-moving and complex regulatory frameworks across geographies.
Regulating AI within the context of privacy can be challenging when data minimization provisions exist within various frameworks, and often requires organizations to examine AI risks through the lens of data governance. This can manifest with AI use cases that demonstrate tangible benefits for the organization, but that do not convert past the proof-of-concept stage due to uncertainty about the risks of AI, both internal and external.
Through applying an end-to-end approach to controlling the risks of AI and right-sizing it for the organization, we’ve helped a range of clients in financial services to gain a full understanding of the risks and scope of their AI deployments.
Policy & principles: Organizations should define a clear set of guiding principles that set the tone from the top. Internally, this means ensuring responsible development, deployment, and use of AI. Externally, the current, and emerging scope of regulatory obligations must be considered to build trust with stakeholders and clients.
Roles & responsibilities: Organizations should establish roles and responsibilities that create oversight through clear visibility and accountability. This includes accountability for the entire AI lifecycle activities, i.e., roles related to approving, reviewing, and monitoring AI-related processes, and driving oversight through clear success metrics. Training and education should be provided to all employees to raise awareness of risks and implications of AI and promote best practices for risk management and incident response.
Risk assessment: Organizations should create a comprehensive system to fully understand, assess, and manage risks related to AI. AI can have knock-on impacts across different environments, including cybersecurity, privacy, operational resilience, data, and financial crime. Risk functions must evolve to effectively manage AI risks, in parallel with implementing the proper controls and understanding the rapidly evolving regulatory obligations. From an operational standpoint, identity and access management should be enhanced to protect highly privileged infrastructure such as trading and market data. AI trading algorithms should be validated through recurring stress testing to assess resiliency against attacks and unexpected events. Business continuity procedures should include redundancy and disaster recovery plans across people, process and technology to ensure continuity and swiftly recover from any failures or disruptions. Firms need to integrate AI risk with information, data and privacy risk management practices, to recognize the overlap of key risks and to ensure coverage.
Lifecycle controls & monitoring: Organizations should fully consider and manage privacy, security, conduct, and resilience risks stemming from the lifecycle of AI development. Robust data governance practices should be implemented throughout to ensure ethical considerations, documentation, and bias mitigation, are properly incorporated. This can directly tie in with the Policy & Principles section above by improving trust in AI model decisions through having transparent practices and employing continuous process validation.
Stakeholder engagement: Organizations should identify key stakeholders to fully realize AI policies and principles. This can start from the top with a corporate acceptable use policy. A proper communication strategy, including notifications and disclosures, based on roles and responsibilities, is necessary to raise awareness while upskilling the entire firm at the same time.
Clear governance of AI enables organizations to gain a deep understanding of their AI-related risks and build the capability to react with agility to regulatory developments. By minimizing the risks and uncertainty in the organization’s governance of AI, it can increasingly be deployed at scale, helping to realize transformational benefits, building data and digital capability, cutting costs, and improving the quality of customer outcomes.
If you'd like to explore these recommendations further, please connect with Brad O'Brien, Lisa Toth, or Son Huynh.
Sources
[1] Federal Bureau of Investigation – Internet Crime Report 2023. https://www.ic3.gov/Media/PDF/AnnualReport/2023_IC3Report.pdf
[2] States are working on AI, but some officials say privacy should come first. https://statescoop.com/state-government-generative-ai-privacy-2024/
[3] Fact Sheet: Vice President Harris Announces OMB Policy to Advance Governance, Innovation, and Risk Management in Federal Agencies’ Use of Artificial Intelligence. www.whitehouse.gov/briefing-room/statements-releases/2024/03/28/fact-sheet-vice-president-harris-announces-omb-policy-to-advance-governance-innovation-and-risk-management-in-federal-agencies-use-of-artificial-intelligence
[4] New draft bipartisan US federal privacy bill unveiled. https://iapp.org/news/a/new-draft-bipartisan-us-federal-privacy-bill-unveiled/
Our Experts
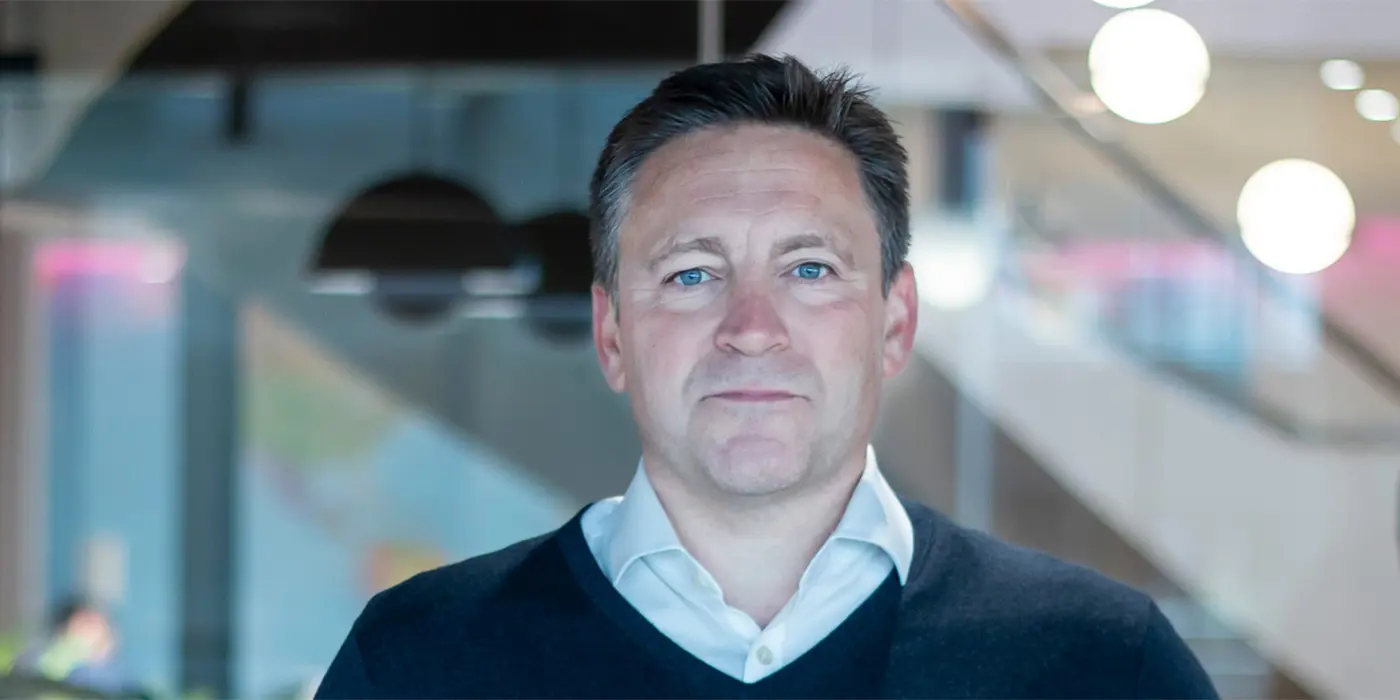
Related Insights
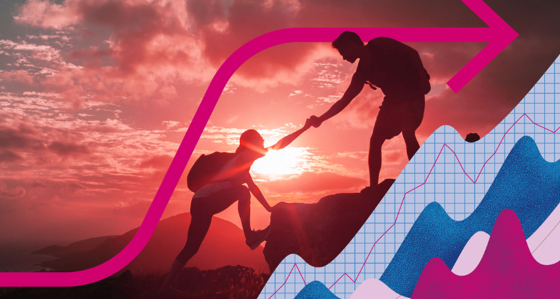
How lenders can scale the refinancing cliff
We surveyed 500 CFOs, financial directors, and senior treasurers in the US and UK to uncover how prepared businesses are to scale the refinancing cliff.
Read more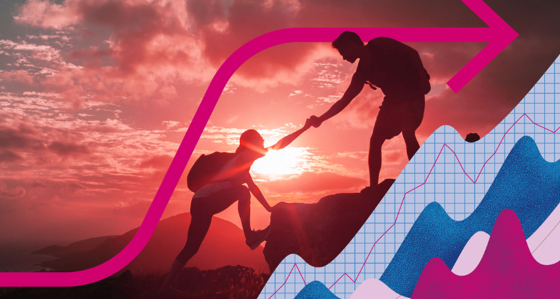
The refinancing cliff: what it is, how it affects you, and how to survive it
The world is facing a “refinancing cliff”, with businesses paying billions of additional dollars per year due to the effect of higher interest rates. But few of the senior execs charged with coordinating their firms’ responses have professional experience of such a macroeconomic environment.
Read more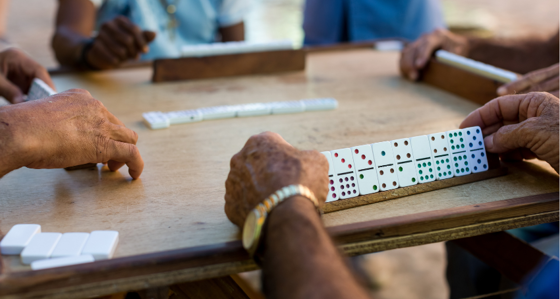
When risks converge, do you know how your financial dominoes will play out?
Addressing the interconnection between risks isn’t just prudent; it's essential for firms to safeguard their financial stability and remain resilient in an increasingly unpredictable world. But how can organisations go about building that all-important view of interconnecting risks and their potential impacts?
Read more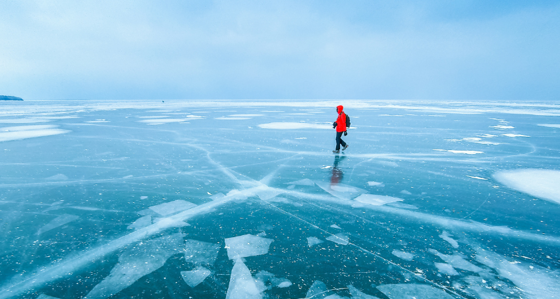
Tackling your emerging risks
No one can know for certain what the future holds. But that’s no excuse for being unprepared when the worst does happen. We uncover how to build strong defences against emerging risks.
Read moreRelated Case Studies

Streamlining home insurance enables reducing operating costs
How do you deliver digital transformation with more certainty, fewer delays and minimal disruption - at a pace that defies conventional expectations?
Read more
Future proofing ESG materiality assessments for a global insurer
How do you prepare now, to stay ahead of changing disclosure requirements?
Read more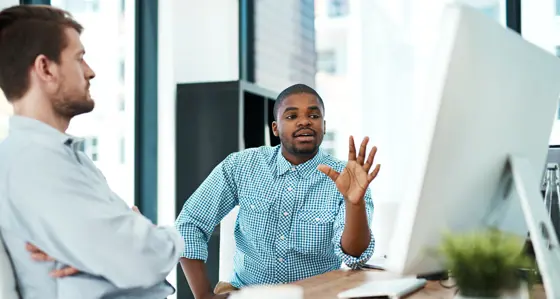
Supporting reluctant customers to become a digital-first insurer
How did a leading healthcare cover provider overcome initial resistance from customers to encourage them to embrace new digital channels?
Read more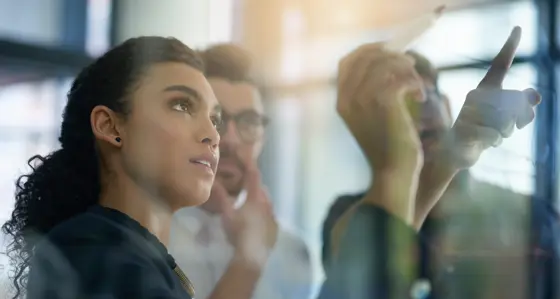
Leading the operational transformation that made a £4 billion insurance deal possible
How did an insurer cut through the complications of one of the biggest carve-outs in recent history to emerge stronger and better equipped for the future?
Read more