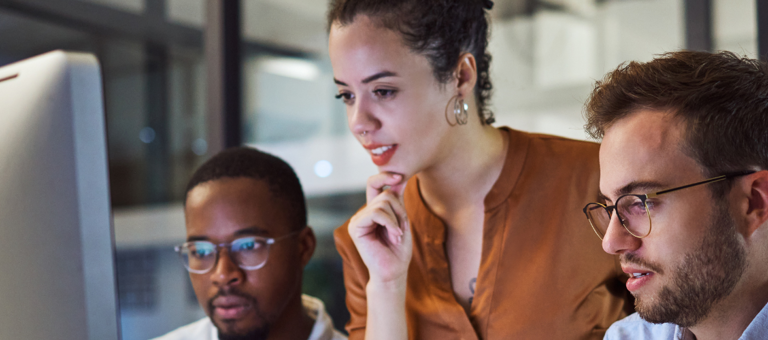
How a different approach to AI can help financial institutions realize its promise
5 min read 16 April 2025
The financial services industry is awash with AI buzzwords. Yet, many institutions struggle to translate AI's potential into tangible, bottom-line impact. Pilot projects sputter, proof of concepts remain just that, and the transformative power of AI often remains elusive. This gap stems from a critical disconnect: the lack of a structured, data-driven approach to identifying and developing truly impactful AI solutions. This article outlines a pragmatic framework for overcoming this challenge, focusing on a "data-driven discovery" model that empowers businesses to identify and capitalize on the most valuable AI opportunities.
The AI opportunity: navigating the hype and overcoming the challenges
Successfully implementing AI within the financial services sector requires navigating a complex landscape of challenges. These include:
Data challenges
Accessing and integrating high-quality data across disparate systems remains a significant obstacle.
Prioritized investments in "shiny objects"
A focus on flashy AI applications like chatbots often overshadows more impactful, yet less visible, opportunities.
Legacy infrastructure and “technical debt”
Existing systems can hinder the seamless integration of AI solutions.
AI skills gap
Concentrating AI expertise in centralized teams limits accessibility and can be inefficient.
Satisfying internal risk and governance concerns
Establishing robust frameworks and controls to address potential risks associated with AI models.
Regulatory complexity
Navigating the evolving regulatory landscape surrounding AI requires careful consideration.
To successfully leverage AI, organizations must focus on business value
True AI success hinges on prioritizing business value over technology. To be successful, financial institutions need to consider:
- Business-driven tailoring
Each AI initiative must be meticulously aligned with specific, quantifiable business objectives. - A data-centric approach
Prioritize data quality, accessibility, and integration across systems. - Explainability and interpretability
Ensure transparency and compliance in AI model decision-making. - Human-in-the-loop
Integrate human oversight to enhance AI model performance and build trust. - Rigorous ROI evaluation
Define and track KPIs to measure the impact of AI investments.
What’s more, successful AI adoption often involves augmenting existing processes rather than completely overhauling them. For example, instead of replacing an entire loan origination process, AI can be used to automate specific tasks like document verification, fraud detection, and risk assessment, leading to increased efficiency and improved customer experience.
How are financial services institutions finding success with AI?
Several leading financial institutions are already demonstrating successful AI adoption strategies through different approaches.
Some organizations, such as BlackRock, are leveraging plug-and-play solutions by utilizing pre-built AI tools and applications like Microsoft 365 Copilot, for immediate use. This fosters widespread access and can stimulate broader interest in AI applications.
Others are focusing on exploring the potential of AI using of APIs and by customizing open-source models to integrate into existing workflows. Wells Fargo’s virtual assistant, "Fargo," which utilizes Google’s PaLM 2 LLM, is a prime example, providing customers with personalized banking insights and executing tasks like checking credit scores and paying bills.
Meanwhile, some financial institutions are establishing AI Centers of Excellence to help build and maintain internal AI products across their organizations. J.P. Morgan’s XAI CoE, for instance, focuses on explainable and fair AI, bringing together experts to develop and share tools and research.
These examples demonstrate different approaches to AI adoption based on an organization's specific needs and objectives.
Introducing the "AI Business Innovation Unit" - a data-driven discovery model to realize the promise of AI
Traditional approaches to AI implementation often follow a "push" model, where a decision is usually made on what use case to spend the investment of AI or Gen AI on – it is a solution looking for a problem. This can lead to a focus on individual department needs or the pursuit of "shiny object" technologies rather than a holistic view of organizational AI potential.
Figure 1 - Traditional "Push" Model
The "AI Business Innovation Hub" flips this model on its head, adopting a "pull" model that focuses on responding to specific business demands. The Hub acts as a centralized structure, receiving requests from business units for solutions to their challenges. The Hub then critically assesses these requests, determining whether an AI-based solution is the most appropriate and feasible approach through not only a thorough understanding of the business process in question but also the input and output data associated with the process (availability, quality, sufficiency of data). This ensures that AI initiatives are directly aligned with genuine business needs and priorities.
Figure 2 - The New "Pull" Model
This approach allows for a more rigorous evaluation of potential AI applications. Before embarking on any initiative, the Hub establishes clear criteria for assessing feasibility, including:
- Business value assessment: Is the potential business impact significant and measurable?
- Data availability and quality: Is sufficient high-quality data available to support the development and deployment of an effective AI model?
- Technical feasibility: Can the proposed AI solution be technically implemented within the existing infrastructure and with available resources?
This rigorous upfront assessment ensures that only the most promising AI initiatives are pursued, maximizing the return on investment and minimizing the risk of failed projects. Furthermore, instead of a “big bang” approach to full scale operationalization, the approach adopts a “proof-of-concept" approach to verify and validate the solution before fully industrializing and productionizing it.
The Hub itself is structured into distinct "pods" to facilitate efficient collaboration and specialization. These include:
- Intake and liaison: Receives and evaluates business requests, acting as liaisons between business units and the Hub.
- Business process and data assessment: Investigates business processes, identifies pain points, and assesses data availability.
- Solution design and planning: Translates business requirements into technical specifications and develops detailed project plans.
- Implementation and delivery: Develops, deploys, and maintains AI models, with ongoing monitoring and evaluation on a smaller scale and scope as a “proof-of-concept.”
Figure 3 - AI Business Innovation Unit
This structured approach fosters cross-functional collaboration and accelerates the development and deployment of high-impact AI solutions.
Empowering AI-driven transformation
Successfully implementing an AI Business Innovation Hub requires a deep understanding of the financial services landscape, durable foundation in data management and AI technologies and the ability to navigate complex organizational change. Baringa has deep expertise in these three areas, having assisted numerous financial institutions in navigating the complexities of AI adoption. Our experience spans designing and implementing AI Business Innovation Units; identifying and prioritizing high-impact AI use cases; developing and deploying robust AI solutions; and building a data-driven culture.
To unlock the full potential of AI, drive innovation, and gain a competitive edge in the rapidly evolving financial services landscape, get in touch with Brad O'Brien, Kaz Kazmi, Merry Spears or Morgan Voytek.
Our Experts
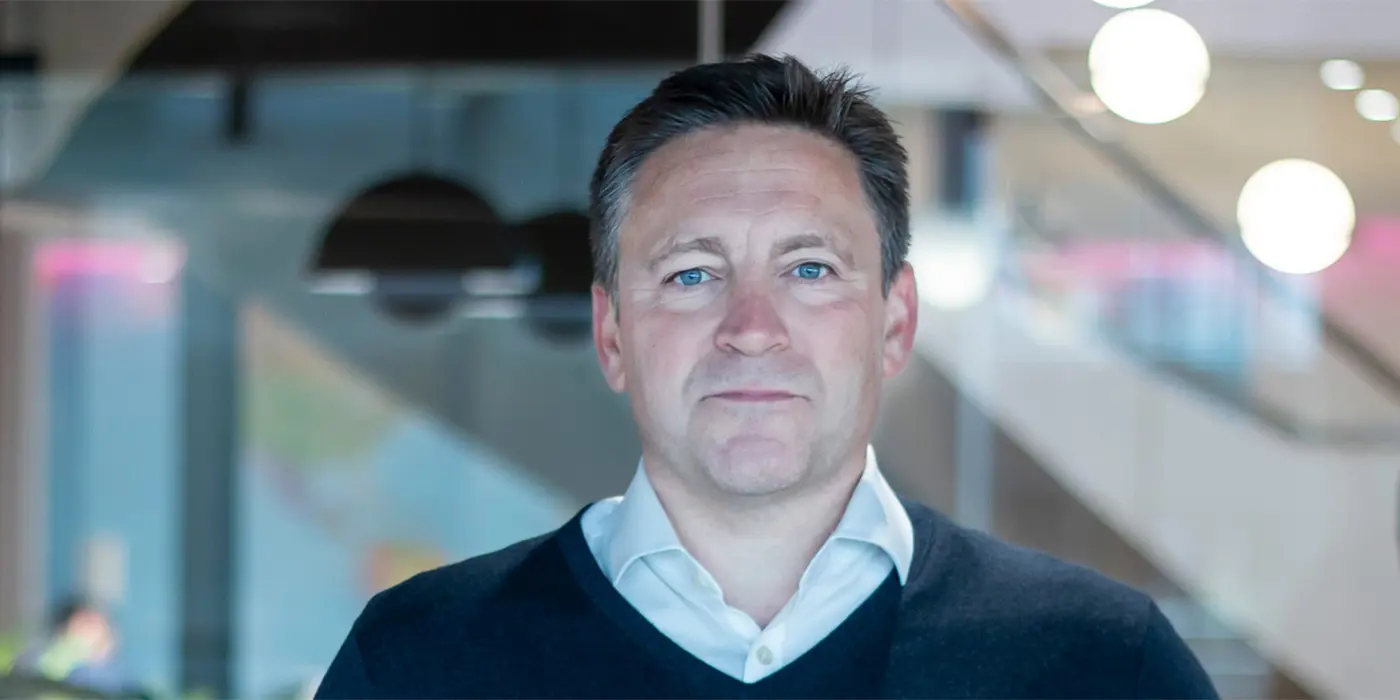
Related Insights
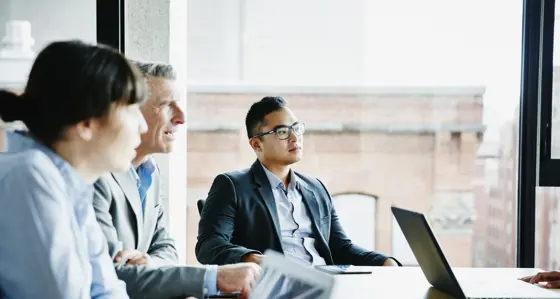
How do super CROs navigate the double-edged sword of AI?
Explore how AI is transforming risk management for superannuation funds and the strategies Chief Risk Officers are using to navigate the complexities of the modern financial landscape.
Read more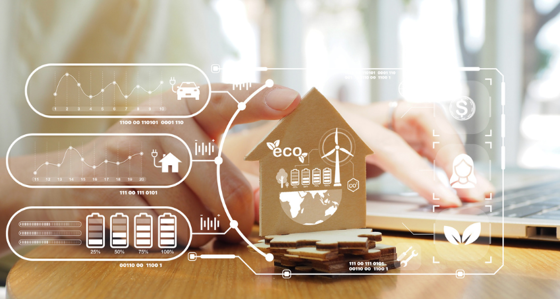
Data-driven decarbonisation: how analytics and AI are supporting the energy transition
Data and AI are key to enabling mass-market decarbonisation. From standardisation to sharing, everyone has a role in ensuring their approach delivers industry-wide benefits alongside individual competitive advantages.
Read more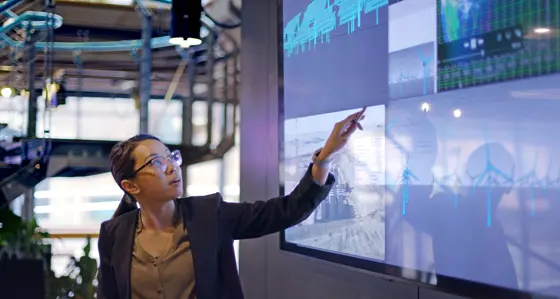
The role of digital technology in the energy transition
With so much at stake, we see four digital technologies standing out as key.
Read more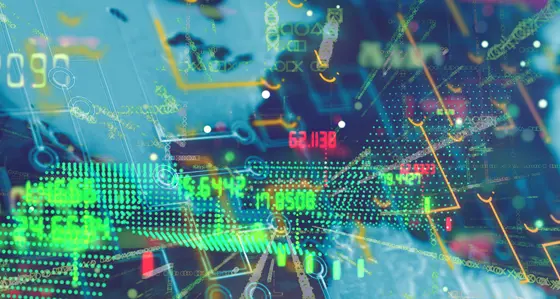
Leadership Dialogues: Data management and workflow solutions
In this video, Baringa's Lucine Tatulian speaks to Roy Saadon, CEO and Co-Founder of Access Fintech about data management and workflow solutions.
Read moreIs digital and AI delivering what your business needs?
Digital and AI can solve your toughest challenges and elevate your business performance. But success isn’t always straightforward. Where can you unlock opportunity? And what does it take to set the foundation for lasting success?