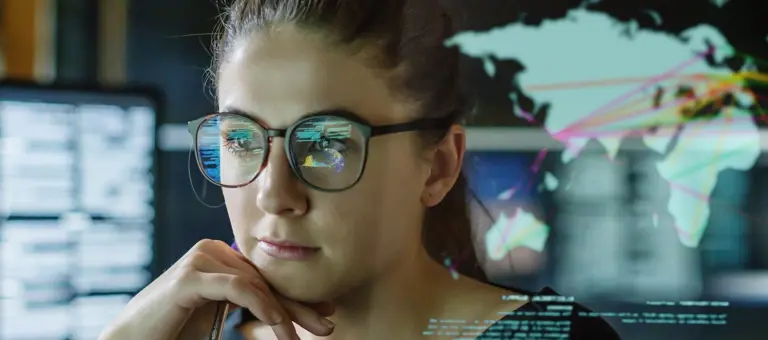
Customer screening: challenges and potential solutions
1 November 2021
To achieve confidence in the effectiveness of customer screening, as well as to minimise waste in the process, there a number of common challenges that should be addressed. In this article we review these challenges and outline potential solutions to overcome them.
Customer Screening Challenges
A number of common challenges exist when using screening to identify sanctioned and high-risk customers.
(Click image to expand)
- Policy does not clearly articulate screening risk appetite: Definitions of PEPs and special interest individuals or entities of interest to the bank are not elaborated in Policy. Investigators have little guidance when determining if a true match is relevant for the bank, and the course of action that should be taken.
- Poor customer data quality/completeness: Insufficient or inaccurate data held on customers to uniquely identify them. For example, a missing or incomplete date of birth field significantly reduces the information available to determine potential matches against watch lists by the screening engine. This results in a long list of potential matches, that could otherwise have been excluded using date of birth.
- Watch lists contain many spurious entries: Third party watch lists are not tailored to organisations’ risk appetites, leading to significant over-screening. Less than 2% of watch list entries relate to sanctions, with the remaining 98% being subject to risk appetite: PEPs, relatives and close associates and special interest individuals/entities, all of which may not be relevant to a particular organisation.
- Poor watch list data quality: Poor data quality in lists can lead to missed true matches and/or additional false matches (where the system could not rule out potential matches).
- Matching logic is not fit-for-purpose, for example:
- Being too strict (in order to reduce alert volumes), leading to missed alerts and the organisation being unable to manage associated financial crime risk effectively
- Being too loose (to compensate for data quality issues), leading to many false positive alerts and a high associated operational cost
- Misalignment with the customer and watch list data model and data quality – e.g. ‘Date of Birth’ field is used for matching but ‘Year of Birth’ is not used in cases where only the year is known
- Investigation procedures are not robust: For example, the permitted fields for discounting an alert or the use of open-source data are not clearly defined
Potential solutions
There is no one size fits all solution to these issues. Point solutions are required for customer data quality issues, matching logic and investigation procedures, with Baringa having significant experience supporting clients in these areas.
For the remaining challenges we have developed a solution to support banks make watch lists fit for purpose- our watch list optimisation tool. The image below shows how our watch list optimisation tools fits into the customer screening process, as well as where other common industry solutions can be leveraged to improve the effectiveness and efficiency of customer screening.
(Click image to expand)
The solutions of watch list optimisation, secondary screening and alert decisioning seen in the diagram above can be used in isolation or in combination to improve the customer screening process. We believe however that watch list optimisation offers significant benefits over secondary screening and auto-decision solutions – the reasoning behind this can be found below.
Watch list optimisation
Watch list is tailored to risk appetite prior to screening to ensure alerts are generated for individuals and entities which pose a financial crime risk to the organisation.
PROS | CONS |
Alerts relating to parties which are known not to be of interest to the organisation will never be generated | More detailed consideration needs to be given to risk appetite upfront |
By enriching data quality prior to screening, the proprietary name screening algorithms will still be utilised, leading to more accurate matches and reduction in false negatives | |
Low integration effort as it does not require changes to the watch list configuration or name screening systems | |
Easily configurable to rapidly adjust to changes in risk appetite and / or policy |
Secondary screening
Additional logic is applied to prioritise alerts according to the risk and / or match strength associated with an alert, potentially enabling auto-closure.
PROS | CONS |
May be able to enhance matching algorithms utilised by the name screening system if they are poorly configured or inadequate | Alerts which are low likelihood but high risk may be de-prioritised (e.g. a valid match on an individual such as Alan Smith may be de-prioritised because the likelihood of a true match is low, due to name commonality) |
Creates redundancy in terms of systems used to determine if an alert is produced and leads to significant maintenance challenges. Should the primary screening engine be modified, secondary screening will need to be re-tuned |
Auto-decisioning
Robotic Process Automation (RPA) or Machine learning models are used to simulate investigator decisions, potentially enabling auto-closure.
Eliminates manual effort of rules-based decisions made by investigators, allowing them to focus on alerts which are more open to interpretation |
Without considering the underlying lists in detail, may not be able to discount alerts relating to spurious list entries |
|
Significant verification of model required to ensure auto-decisioning is not closing alerts featuring genuine risk exposure |
PROS |
CONS |
If you would like to know more about our Watch List Optimisation solution and how it could benefit your organisation, please contact Cat Morris.
Related Insights
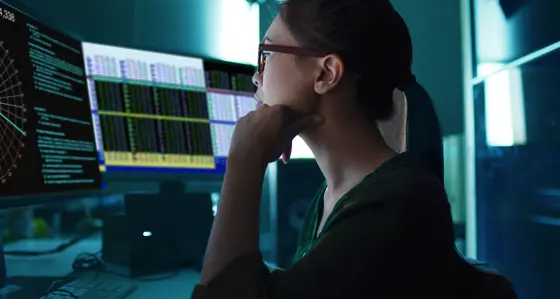
Typify™ - more accurately detecting Financial Crime
Typify, Baringa's investigation and analytics product, creates a fit for purpose solution that is easy to use and implement.
Read more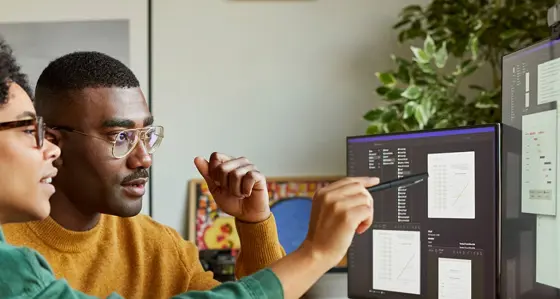
An introduction to Typify™
Typify™accurately pinpoints the atypical by combining an understanding of typical customer and peer group behaviour with insight from financial crime experts. This enables financial institutions to manage financial crime effectively and efficiently, reducing the need for large and expensive teams of investigators.
Read more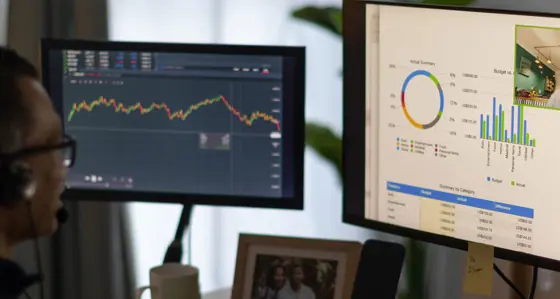
Typify™ - financial crime typologies identified
Transaction monitoring is key to detecting suspicious financial activity. However, this is not 100% accurate. Baringa’s Typify offers a tailored solution.
Read more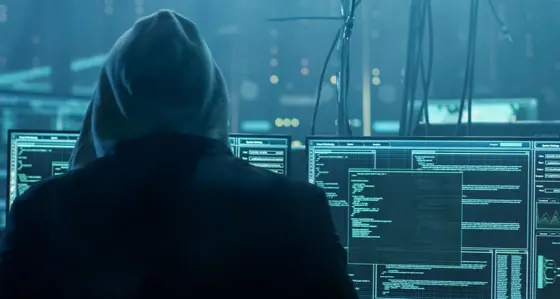
Top 10 financial crime risks 2022
Baringa is committed to reducing financial crime and the negative consequences it has on society.
Read moreAre digital and AI delivering what your business needs?
Digital and AI can solve your toughest challenges and elevate your business performance. But success isn’t always straightforward. Where can you unlock opportunity? And what does it take to set the foundation for lasting success?