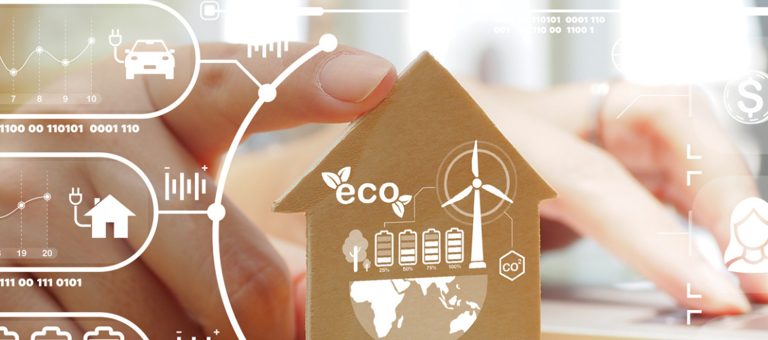
Data-driven decarbonisation: how analytics and AI are supporting the energy transition
8 min read 22 July 2024
At Baringa’s fifth Green Buildings and Transport Network roundtable in June 2024, we focused on a headline-grabbing topic: data and AI.
We know these must play a key role in enabling asset, home, and grid optimisation; bringing together fragmented pieces of the ‘energy transition’ puzzle; and helping consumers take ownership of their energy.
Those are lofty ambitions that will take time to achieve. So where is the market currently? And what progress are organisations making in leveraging data and AI? Our vibrant discussion covered these key questions.
It’s still early days, but we’re at a critical point in the data and AI journey
Industry players are increasingly using data and AI to enhance customer propositions, reduce costs, and streamline operations. As Costas Gavriel from Baringa’s Data Analytics & AI team said, ‘We’re at the point where data is readily available, sophisticated models mean you don’t need an army of data scientists, and computing power is cheap and accessible. The next step is understanding how to drive more intelligent analysis to enable more intelligent decision-making.’
To develop this understanding, organisations are taking different approaches. Our discussion clearly showed that smart energy infrastructure and software providers are further along the journey than financial services, which have a more constrained regulatory environment. However, there was a clear consensus that data and AI will be essential to driving the energy transition. As Patrick Caiger-Smith, chairman of Green Energy Options (GEO), commented: ‘It’s still early days, and commercial deployability is limited. But if you’re not actively exploring how to leverage and optimise AI, you will be behind the curve within two years.’
How data and AI are starting to transform operations and drive insights
Our roundtable participants discussed a range of data and AI use cases their organisations are actively implementing. The highlights related to:
- quantifying the value of – and demand for – green homes
- taking a whole-house approach to optimisation
- managing pressure on the grid
- predicting customer energy demand
- providing personalised energy saving recommendations
- increasing the efficiency and quality of smart meter installations
- improving the customer experience.
Quantifying the value of – and demand for – green homes
Timothy Bannister, director of property design and data at Rightmove, shared fascinating insight into how Rightmove is using its wealth of consumer behaviour and property data. By taking a creative approach to analysis, they can quantify the value of low-carbon technology in homes and track trends around demand.
Their analyses show a growing awareness of low-carbon tech benefits, which is increasingly reflected in people’s approach to buying and renting. For instance:
- They calculated a 15% value uplift for homes that upgraded from EPC F to EPC C
- ‘Solar panels’ is now in the top 100 most-searched keywords on Rightmove, up from the top 500 in 2020. ‘Heat pumps’ has also surged from >1,000th place in 2020 to the top 200 in 2024.
Taking a whole-house approach to optimisation
Whole-house optimisation has enormous potential to drive savings for consumers (through lower energy bills) and value for energy suppliers (by increasing their margins).
Patrick Caiger-Smith talked about a GEO project that uses machine learning to deliver a whole-house approach to optimising dynamic loads from heat pumps, EVs, and solar panels. Through trials, they found that using variable tariffs and battery storage to run systems at optimum times can save homes 50% on energy bills. This whole-house approach has also reduced the home’s carbon footprint by 20%.
Andy Beggs, technical product manager for Smart Metering Systems (SMS), also discussed their work for a government contract on using energy smart appliances and communication protocols. This would allow AI to facilitate automatic load optimisation based on a dynamic picture, considering for variables like time of day and weather conditions.
Managing pressure on the grid
Patrick from GEO also discussed a significant project with trade association BEAMA and Energy Systems Catapult. At present, it’s hard for distribution network operators (DNOs) to understand the impact of increased electrification on local networks. This project involved modelling at the distribution centre level to understand the best ways to reduce grid reinforcement requirements. For example, what level of storage do homes need? How do we maximise cable and feeder use to avoid having to dig up roads? And how can we automate assets to increase capacity and lifespan while minimising disruption?
Baringa is also working on a live trial with DNOs and energy suppliers around optimising EV charging. Machine learning models calculate half-hourly pricing based on demand and pressure on the local station with the aim being to enable collaboration so suppliers can optimise costs and DNOs can manage demand – while ensuring customers’ EVs are charged to the required amount by a specified time.
Predicting customer energy demand
Alex Schoch, who leads Octopus Energy’s flexibility and electrification program, discussed an interesting virtual power plant project. Using a sophisticated regression model, Octopus can forecast how many cars will be plugging in per day, what time they’ll plug in, how much charge they’ll need, and what the vehicle settings will be. They’ve achieved greater than 90% accuracy and enabled customers to save about £500 on energy for EV charging. It’s a great example of a commercially viable use case that provides a material benefit to customers.
Providing personalised energy saving recommendations
SMS and Eliq are among the partners working with Oxfordshire County Council on the Energy Saver App. This new free app uses smart meter data and AI to give residents personalised, actionable tips for reducing energy use.
Increasing the efficiency and quality of smart meter installations
Andrew Beggs from SMS mentioned an interesting AI application that helps their engineers install and commission equipment. They’ve introduced bots on handheld terminals, which guide engineers through processes and help with troubleshooting.
Improving the customer experience
Since April 2023, Octopus Energy has augmented its customer messaging platform with generative AI. Capabilities include summarising customer interactions for agents, extracting highlights from recorded phone calls, and creating first drafts of messages in response to queries.
40% of customer interactions at Octopus Energy UK are now augmented with generative AI – with impressive results. The AI-augmented messages have a 19.5% higher customer happiness index score than messages written manually.
How to enable industry-wide collaboration that drives broad adoption
These were great examples of where organisations are starting to innovate with data and AI. But how do we unlock value on a wider scale?
Our attendees had interesting ideas around:
- building consumer awareness and trust
- experimenting with available data
- building in testing and feedback
- focusing on interoperability and data sharing.
Start by building consumer awareness and trust
A key barrier to domestic decarbonisation is the gap in customer engagement and understanding. Therefore, a good starting point involves meeting customers where they are; catering to their current mindset and pain points; and keeping things simple.
For example, Daniel Anderton, senior data scientist at Eliq, discussed how they are delivering personalised energy saving insights at scale. Using a home’s energy consumption data along with historical data, their solution uses a relevance engine to extract the best energy saving tips from an extensive advice catalogue. Eliq has seen a 7% year-on-year reduction in energy consumption for users just from increasing their understanding of quick wins.
These incremental achievements drive awareness and engagement. They also build trust in AI-based solutions, which lays a crucial foundation for when more sophisticated personalisation, locational pricing, and dynamic tariffs become possible.
Experiment with what’s available – don’t wait for better data
Even though the smart meter roll-out is taking longer than many would like and Open Energy isn’t quite there, valuable data is available to leverage. For example, N3rgy.com provides access to smart meter data with customer consent – without having to go through energy suppliers. You can use this to start understanding energy costs, optimising operations, and improving forecasting, as well as creating compelling customer propositions.
Build in testing and feedback
Timothy Bannister from Rightmove summed this up nicely: ‘There are some areas where AI is interesting and some where it’s valuable. We have to target the areas where it’s valuable, not just interesting. And that must involve continuous testing.’
It’s imperative that organisations build feedback into their strategies and projects. It’s not just about insights into what people can do (to save money or balance loads, for example). It’s also about insights into what’s happening and feeding that back in. This is a crucial piece of the mass adoption puzzle because it ensures we’re constantly challenging assumptions and learning from evolving behaviour.
Focus on interoperability and data sharing
Costas Gavriel from Baringa talked about how ‘AI is at the point where it’s good at solving narrow scoped problems. However, in energy industry, we can have different providers trying to optimise different aspects that can contradict with each over: DNOs are trying to optimise load, and energy suppliers are trying to manage customer costs through TOU tariffs. If those people aren’t sharing information and working together, problems can arise.’
Therefore, interoperability and data standardisation are essential. Progress has been made in this area – for example, through the EEBUS and Matter communication protocols. But unless organisations prioritise data sharing and communication, it will be difficult to drive mass adoption.
Leveraging data and AI requires ongoing coordination across the ecosystem
Data and AI are key to enabling mass-market decarbonisation. And that requires ongoing progress to unify and coordinate the energy data landscape. From standardisation to sharing, everyone has a role in ensuring their approach delivers industry-wide benefits alongside individual competitive advantages. Because this isn’t a zero-sum game – it’s one we all win together.
Want to discover how we can help you? Explore our Data and AI consulting services
Our Experts
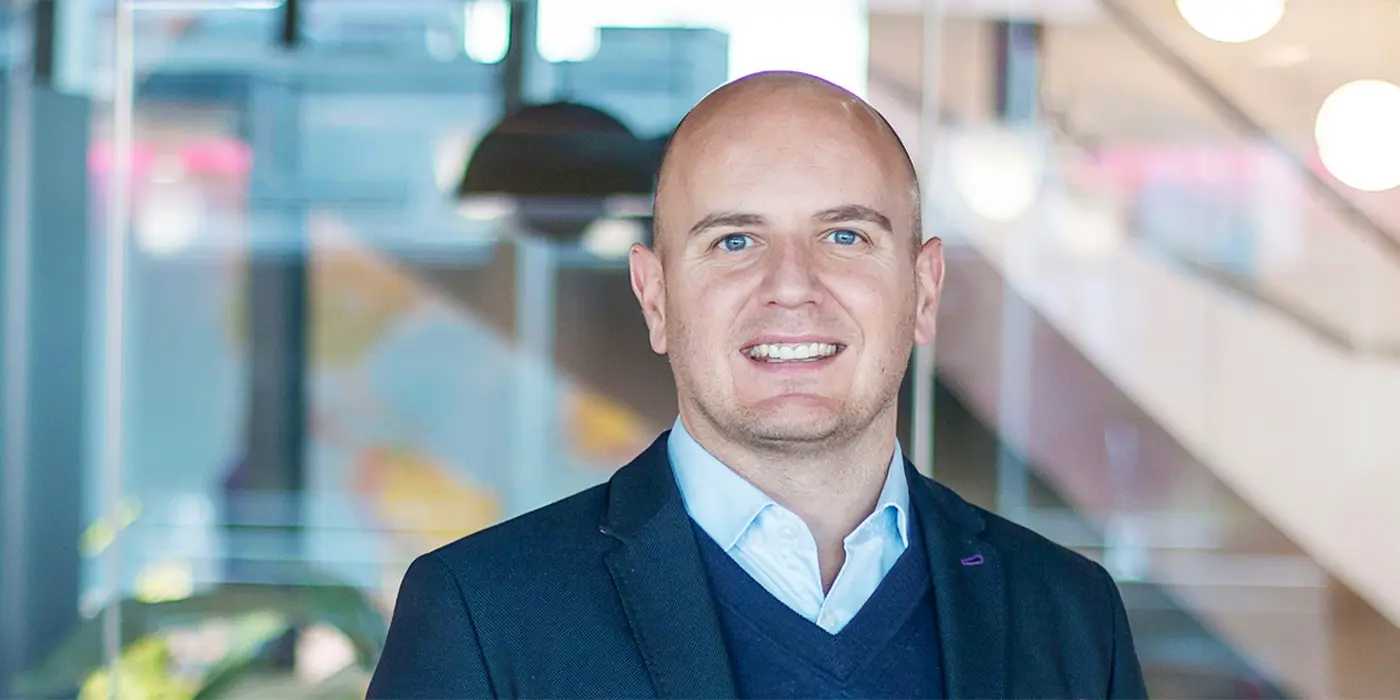
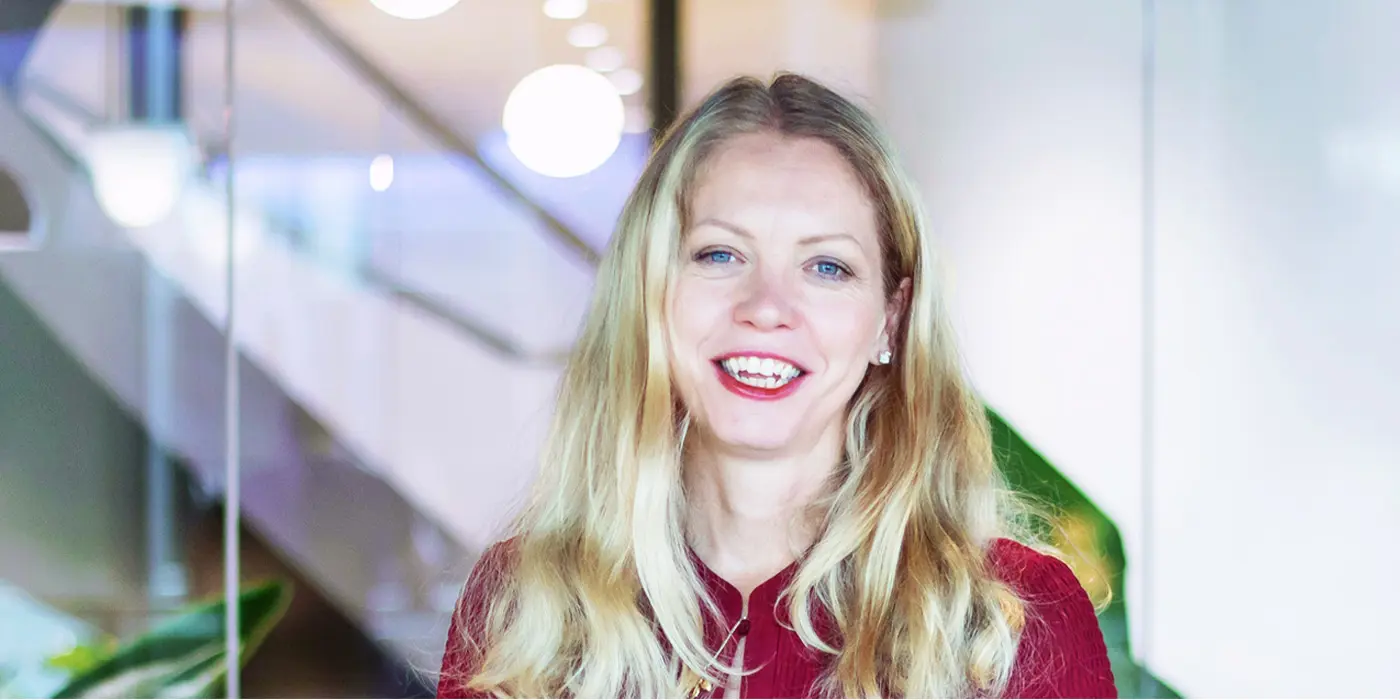
Related Insights
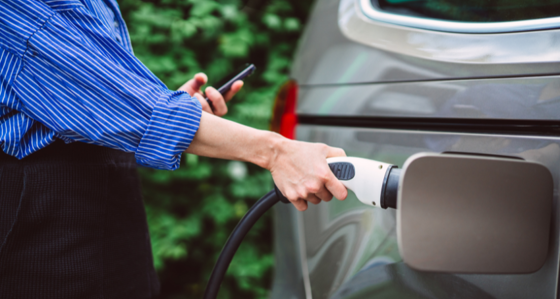
Trump trade tariffs: Impact on the UK EV uptake
The global automative industry is at a critical juncture, shaped by intersecting forces of electrification, geopolitical trade tensions, and national decarbonisation policy.
Read more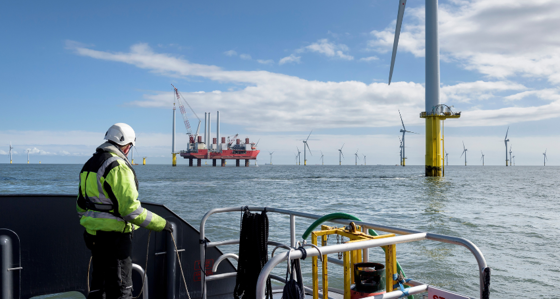
Driving economic growth by securing a cleaner and resilient future for the North Sea
Our report, published in conjunction with Offshore Energies UK (OEUK), outlines how the North Sea can continue to play a pivotal role in the energy system as the world shifts to net zero, while positioning the UK as a resilient, innovative and competitive player in the global energy landscape.
Read more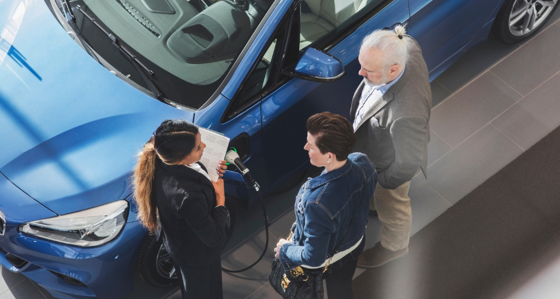
From early adopters to early majority: Driving the next phase of EV growth
As EVs are moving beyond the early adopter phase, what are the principles needed for mass market adoption and progressing towards net zero targets?
Read more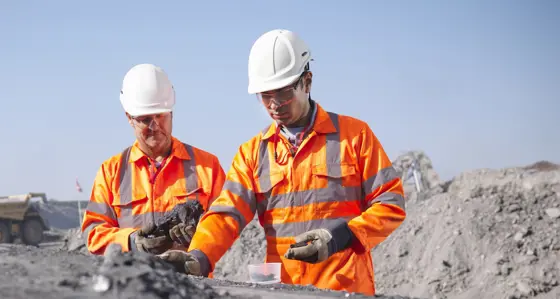
Crossing the industry divide to drive decarbonisation across Asia-Pacific
Resources organisations are identifying opportunities in the unfamiliar overlap between energy, mining and metals.
Read moreRelated Client Stories
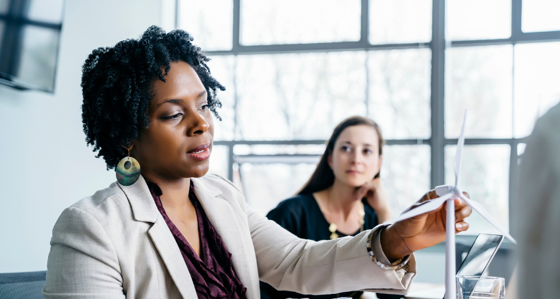
Maximising impact from AI investment for a global energy leader
Discover how a series of experiential learning events resulted in employee confidence in AI skills hitting 85%.
Read more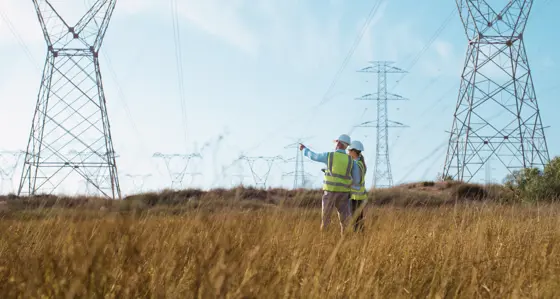
Maintaining the stability of the New South Wales power system
How do you ensure the future stability of the NSW power system?
Read more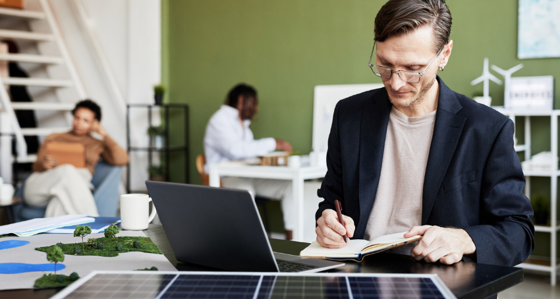
Using AI to manage applications for connecting low-carbon devices
With growing calls for processes to be standardised, Baringa and Energy Networks Association (ENA) came together to develop and launch the first central industry platform to transform the way registrations for low-carbon devices are managed.
Read more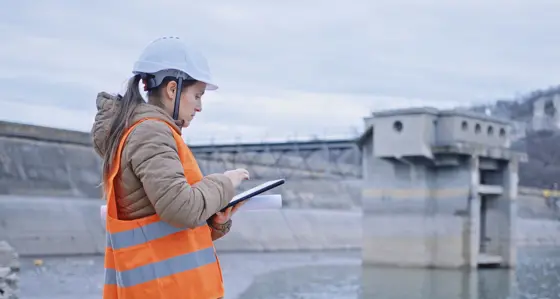
Collaborating globally to future proof Australia’s water resources
How do you effectively manage the ever-evolving supply and demand challenges of water resources?
Read moreIs digital and AI delivering what your business needs?
Digital and AI can solve your toughest challenges and elevate your business performance. But success isn’t always straightforward. Where can you unlock opportunity? And what does it take to set the foundation for lasting success?